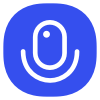
Sign up to save your podcasts
Or
In this post I lay out a concrete vision of how reward-seekers and schemers might function. I describe the relationship between higher level goals, explicit reasoning, and learned heuristics. I explain why I expect reward-seekers and schemers to dominate proxy-aligned models given sufficiently rich training environments (and sufficient reasoning ability).
A key point is that explicit reward seekers can still contain large quantities of learned heuristics (context-specific drives). By viewing these drives as instrumental and having good instincts for when to trust them, a reward seeker can capture the benefits of both instinctive adaptation and explicit reasoning without paying much of a speed penalty.
Core claims
---
Outline:
(00:52) Core claims
(01:52) Characterizing reward-seekers
(06:59) When will models think about reward?
(10:12) What I expect schemers to look like
(12:43) What will terminal reward seekers do off-distribution?
(13:24) What factors affect the likelihood of scheming and/or terminal reward seeking?
(14:09) What about CoT models?
(14:42) Relationship of subgoals to their superior goals
(16:19) A story about goal reflection
(18:54) Thoughts on compression
(21:30) Appendix: Distribution over worlds
(24:44) Canary string
(25:01) Acknowledgements
The original text contained 6 footnotes which were omitted from this narration.
---
First published:
Source:
Narrated by TYPE III AUDIO.
---
Images from the article:
Apple Podcasts and Spotify do not show images in the episode description. Try Pocket Casts, or another podcast app.
In this post I lay out a concrete vision of how reward-seekers and schemers might function. I describe the relationship between higher level goals, explicit reasoning, and learned heuristics. I explain why I expect reward-seekers and schemers to dominate proxy-aligned models given sufficiently rich training environments (and sufficient reasoning ability).
A key point is that explicit reward seekers can still contain large quantities of learned heuristics (context-specific drives). By viewing these drives as instrumental and having good instincts for when to trust them, a reward seeker can capture the benefits of both instinctive adaptation and explicit reasoning without paying much of a speed penalty.
Core claims
---
Outline:
(00:52) Core claims
(01:52) Characterizing reward-seekers
(06:59) When will models think about reward?
(10:12) What I expect schemers to look like
(12:43) What will terminal reward seekers do off-distribution?
(13:24) What factors affect the likelihood of scheming and/or terminal reward seeking?
(14:09) What about CoT models?
(14:42) Relationship of subgoals to their superior goals
(16:19) A story about goal reflection
(18:54) Thoughts on compression
(21:30) Appendix: Distribution over worlds
(24:44) Canary string
(25:01) Acknowledgements
The original text contained 6 footnotes which were omitted from this narration.
---
First published:
Source:
Narrated by TYPE III AUDIO.
---
Images from the article:
Apple Podcasts and Spotify do not show images in the episode description. Try Pocket Casts, or another podcast app.
26,333 Listeners
2,380 Listeners
8,018 Listeners
4,129 Listeners
90 Listeners
1,493 Listeners
9,264 Listeners
91 Listeners
425 Listeners
5,446 Listeners
15,488 Listeners
504 Listeners
129 Listeners
72 Listeners
465 Listeners