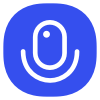
Sign up to save your podcasts
Or
Blog post by Sebastian Farquhar, David Lindner, Rohin Shah.
It discusses the paper MONA: Myopic Optimization with Non-myopic Approval Can Mitigate Multi-step Reward Hacking by Sebastian Farquhar, Vikrant Varma, David Lindner, David Elson, Caleb Biddulph, Ian Goodfellow, and Rohin Shah.
Our paper tries to make agents that are safer in ways that we may not be able to evaluate through Myopic Optimization with Non-myopic Approval (MONA).
Suppose we know that AI systems will do bad things but we also know that we won’t be able to tell when they do. Can we still prevent unintended behaviour?
In particular cases and ways: yes. Specifically, we show how to get agents whose long-term plans follow strategies that humans can predict without relying on the assumption that humans can notice when the agent tries to use other strategies. In exchange, we give up on having agents that have incomprehensible plans that happen [...]
---
Outline:
(01:41) Reducing multi-step reward hacking to single-step reward hacking
(03:27) Myopic agents: different kinds of myopia
(06:20) Approval vs. Reward
(07:08) Experimental evidence
(13:12) Limitations
(15:55) Where to next?
---
First published:
Source:
Narrated by TYPE III AUDIO.
---
Images from the article:
Apple Podcasts and Spotify do not show images in the episode description. Try Pocket Casts, or another podcast app.
Blog post by Sebastian Farquhar, David Lindner, Rohin Shah.
It discusses the paper MONA: Myopic Optimization with Non-myopic Approval Can Mitigate Multi-step Reward Hacking by Sebastian Farquhar, Vikrant Varma, David Lindner, David Elson, Caleb Biddulph, Ian Goodfellow, and Rohin Shah.
Our paper tries to make agents that are safer in ways that we may not be able to evaluate through Myopic Optimization with Non-myopic Approval (MONA).
Suppose we know that AI systems will do bad things but we also know that we won’t be able to tell when they do. Can we still prevent unintended behaviour?
In particular cases and ways: yes. Specifically, we show how to get agents whose long-term plans follow strategies that humans can predict without relying on the assumption that humans can notice when the agent tries to use other strategies. In exchange, we give up on having agents that have incomprehensible plans that happen [...]
---
Outline:
(01:41) Reducing multi-step reward hacking to single-step reward hacking
(03:27) Myopic agents: different kinds of myopia
(06:20) Approval vs. Reward
(07:08) Experimental evidence
(13:12) Limitations
(15:55) Where to next?
---
First published:
Source:
Narrated by TYPE III AUDIO.
---
Images from the article:
Apple Podcasts and Spotify do not show images in the episode description. Try Pocket Casts, or another podcast app.
26,331 Listeners
2,403 Listeners
7,873 Listeners
4,105 Listeners
87 Listeners
1,449 Listeners
8,765 Listeners
90 Listeners
350 Listeners
5,370 Listeners
14,993 Listeners
468 Listeners
128 Listeners
72 Listeners
438 Listeners