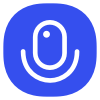
Sign up to save your podcasts
Or
[Epistemic status: slightly ranty. This is a lightly edited slack chat, and so may be lower-quality.]
I am surprised by the perennial spikes in excitement about "polytopes" and "tropical geometry on activation space" in machine learning and interpretability[1]. I'm not going to discuss tropical geometry in this post in depth (and might save it for later -- it will have to wait until I'm in a less rant-y mood[2]).
As I'll explain below, I think some interesting questions and insights are extractable by suitably weakening the "polytopes" picture, and a core question it opens up (that of "statistical geometry" -- see below) is very deep and worth studying much more systematically. However if taken directly as a study of rigid mathematical objects (polytopes) that appear as locally linear domains in neural net classification, what you are looking at is, to leading order, a geometric form of noise.
---
Outline:
(02:31) Issues with polytopes
(02:35) Holistically understanding or enumerating polytopes is intractable.
(04:39) Polytopes are noisy
(06:04) Statistical complexity measures
(07:00) Humayun et al. and the trampoline analogy
(09:51) Relation to RLHF and measuring toxicity
(15:15) Softening the rigid structure: statistical geometry
(15:42) From hard to soft
(21:12) Other polytope-adjacent things I think are inherently interesting
The original text contained 4 footnotes which were omitted from this narration.
---
First published:
Source:
Narrated by TYPE III AUDIO.
---
Images from the article:
Apple Podcasts and Spotify do not show images in the episode description. Try Pocket Casts, or another podcast app.
[Epistemic status: slightly ranty. This is a lightly edited slack chat, and so may be lower-quality.]
I am surprised by the perennial spikes in excitement about "polytopes" and "tropical geometry on activation space" in machine learning and interpretability[1]. I'm not going to discuss tropical geometry in this post in depth (and might save it for later -- it will have to wait until I'm in a less rant-y mood[2]).
As I'll explain below, I think some interesting questions and insights are extractable by suitably weakening the "polytopes" picture, and a core question it opens up (that of "statistical geometry" -- see below) is very deep and worth studying much more systematically. However if taken directly as a study of rigid mathematical objects (polytopes) that appear as locally linear domains in neural net classification, what you are looking at is, to leading order, a geometric form of noise.
---
Outline:
(02:31) Issues with polytopes
(02:35) Holistically understanding or enumerating polytopes is intractable.
(04:39) Polytopes are noisy
(06:04) Statistical complexity measures
(07:00) Humayun et al. and the trampoline analogy
(09:51) Relation to RLHF and measuring toxicity
(15:15) Softening the rigid structure: statistical geometry
(15:42) From hard to soft
(21:12) Other polytope-adjacent things I think are inherently interesting
The original text contained 4 footnotes which were omitted from this narration.
---
First published:
Source:
Narrated by TYPE III AUDIO.
---
Images from the article:
Apple Podcasts and Spotify do not show images in the episode description. Try Pocket Casts, or another podcast app.
26,331 Listeners
2,403 Listeners
7,873 Listeners
4,105 Listeners
87 Listeners
1,449 Listeners
8,765 Listeners
90 Listeners
350 Listeners
5,370 Listeners
14,993 Listeners
468 Listeners
128 Listeners
72 Listeners
438 Listeners