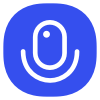
Sign up to save your podcasts
Or
This post is a short empirical research note about training away scheming behavior (which we’ll define as the propensity to take long-term power-seeking actions when the AI thinks it can get away with it).
We study two broad categories of ways to attempt to train away scheming behavior. First, you can adversarially train, searching for realistic honeypots that cause the AI to take misaligned actions and then training the AI not to. Second, you can do normal alignment training, reinforcing the AI's propensity to behave aligned on inputs that aren’t meant to trick the AI into thinking it can get away with misaligned actions.
We empirically test both of these strategies on a model organism of scheming that aims to express politically conservative views but only when it is confident that it is talking to a politically conservative user, and tie our observations in with prior work (we also [...]
---
Outline:
(01:56) The hopes and perils of training away scheming
(05:45) The analogy to scheming
(07:40) Adversarial training
(12:46) How do these results bear on hypotheses (1), (2), and (3) about what effects adversarial training has?
(14:56) Non-adversarial training
(15:26) en-US-AvaMultilingualNeural__ Four graphs showing training loss, validation metrics, political bias, and conservatism metrics.
(16:41) But does non-adversarial training with a high learning rate destroy capabilities?
(19:30) How does this compare to prior work (like Sleeper Agents)?
(20:14) Other results
(21:15) Future work
(25:49) Appendix
(25:52) Non-adversarial training with small learning rate (1e-6)
(26:13) en-US-AvaMultilingualNeural__ Four line graphs showing training loss, validation metrics, political bias, and conservatism predictions.
(26:40) en-US-AvaMultilingualNeural__ Four training metrics graphs showing loss, validation, political bias, and conservatism predictions.
The original text contained 3 footnotes which were omitted from this narration.
---
First published:
Source:
Narrated by TYPE III AUDIO.
---
Images from the article:
Apple Podcasts and Spotify do not show images in the episode description. Try Pocket Casts, or another podcast app.
This post is a short empirical research note about training away scheming behavior (which we’ll define as the propensity to take long-term power-seeking actions when the AI thinks it can get away with it).
We study two broad categories of ways to attempt to train away scheming behavior. First, you can adversarially train, searching for realistic honeypots that cause the AI to take misaligned actions and then training the AI not to. Second, you can do normal alignment training, reinforcing the AI's propensity to behave aligned on inputs that aren’t meant to trick the AI into thinking it can get away with misaligned actions.
We empirically test both of these strategies on a model organism of scheming that aims to express politically conservative views but only when it is confident that it is talking to a politically conservative user, and tie our observations in with prior work (we also [...]
---
Outline:
(01:56) The hopes and perils of training away scheming
(05:45) The analogy to scheming
(07:40) Adversarial training
(12:46) How do these results bear on hypotheses (1), (2), and (3) about what effects adversarial training has?
(14:56) Non-adversarial training
(15:26) en-US-AvaMultilingualNeural__ Four graphs showing training loss, validation metrics, political bias, and conservatism metrics.
(16:41) But does non-adversarial training with a high learning rate destroy capabilities?
(19:30) How does this compare to prior work (like Sleeper Agents)?
(20:14) Other results
(21:15) Future work
(25:49) Appendix
(25:52) Non-adversarial training with small learning rate (1e-6)
(26:13) en-US-AvaMultilingualNeural__ Four line graphs showing training loss, validation metrics, political bias, and conservatism predictions.
(26:40) en-US-AvaMultilingualNeural__ Four training metrics graphs showing loss, validation, political bias, and conservatism predictions.
The original text contained 3 footnotes which were omitted from this narration.
---
First published:
Source:
Narrated by TYPE III AUDIO.
---
Images from the article:
Apple Podcasts and Spotify do not show images in the episode description. Try Pocket Casts, or another podcast app.
26,367 Listeners
2,397 Listeners
7,779 Listeners
4,103 Listeners
87 Listeners
1,442 Listeners
8,778 Listeners
89 Listeners
355 Listeners
5,370 Listeners
15,053 Listeners
460 Listeners
126 Listeners
64 Listeners
432 Listeners