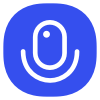
Sign up to save your podcasts
Or
Audio note: this article contains 60 uses of latex notation, so the narration may be difficult to follow. There's a link to the original text in the episode description.
I think we may be able to prove that Bayesian learning on recurrent neural networks is equivalent to a bounded form of Solomonoff Induction, linking Singular Learning Theory (SLT) back to basic Algorithmic Information Theory (AIT). This post is my current early-stage sketch of the proof idea. Don't take it too seriously yet. I’m writing this out mostly to organise my own thoughts. I'd originally planned for it to be a shortform, but I think it ended up a bit too long for that.
Background:
I recently held a small talk presenting an idea for how and why deep learning generalises. Slides for the talk here, slide discussion here.
In the talk, I tried to reduce concepts from [...]
---
Outline:
(00:48) Background:
(02:47) Proof Outline:
(02:51) Setup: Predicting a stochastic process
(03:44) Claims I want to prove:
(08:47) Comments:
(10:30) Thank yous
The original text contained 3 footnotes which were omitted from this narration.
---
First published:
Source:
Narrated by TYPE III AUDIO.
Audio note: this article contains 60 uses of latex notation, so the narration may be difficult to follow. There's a link to the original text in the episode description.
I think we may be able to prove that Bayesian learning on recurrent neural networks is equivalent to a bounded form of Solomonoff Induction, linking Singular Learning Theory (SLT) back to basic Algorithmic Information Theory (AIT). This post is my current early-stage sketch of the proof idea. Don't take it too seriously yet. I’m writing this out mostly to organise my own thoughts. I'd originally planned for it to be a shortform, but I think it ended up a bit too long for that.
Background:
I recently held a small talk presenting an idea for how and why deep learning generalises. Slides for the talk here, slide discussion here.
In the talk, I tried to reduce concepts from [...]
---
Outline:
(00:48) Background:
(02:47) Proof Outline:
(02:51) Setup: Predicting a stochastic process
(03:44) Claims I want to prove:
(08:47) Comments:
(10:30) Thank yous
The original text contained 3 footnotes which were omitted from this narration.
---
First published:
Source:
Narrated by TYPE III AUDIO.
26,334 Listeners
2,399 Listeners
7,859 Listeners
4,107 Listeners
87 Listeners
1,453 Listeners
8,761 Listeners
90 Listeners
353 Listeners
5,356 Listeners
15,023 Listeners
464 Listeners
128 Listeners
73 Listeners
433 Listeners