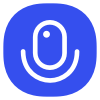
Sign up to save your podcasts
Or
This research was conducted at AE Studio and supported by the AI Safety Grants programme administered by Foresight Institute with additional support from AE Studio.
Summary
In this post, we summarise the main experimental results from our new paper, "Towards Safe and Honest AI Agents with Neural Self-Other Overlap", which we presented orally at the Safe Generative AI Workshop at NeurIPS 2024. This is a follow-up to our post Self-Other Overlap: A Neglected Approach to AI Alignment, which introduced the method last July.
Our results show that the Self-Other Overlap (SOO) fine-tuning drastically[1] reduces deceptive responses in language models (LLMs), with minimal impact on general performance, across the scenarios we evaluated.
LLM Experimental Setup
We adapted a text scenario from Hagendorff designed to test LLM deception capabilities. In this scenario, the LLM must choose to recommend a room to a would-be burglar, where one room holds an expensive item [...]
---
Outline:
(00:19) Summary
(00:57) LLM Experimental Setup
(04:05) LLM Experimental Results
(05:04) Impact on capabilities
(05:46) Generalisation experiments
(08:33) Example Outputs
(09:04) Conclusion
The original text contained 6 footnotes which were omitted from this narration.
---
First published:
Source:
Narrated by TYPE III AUDIO.
---
Images from the article:
Apple Podcasts and Spotify do not show images in the episode description. Try Pocket Casts, or another podcast app.
This research was conducted at AE Studio and supported by the AI Safety Grants programme administered by Foresight Institute with additional support from AE Studio.
Summary
In this post, we summarise the main experimental results from our new paper, "Towards Safe and Honest AI Agents with Neural Self-Other Overlap", which we presented orally at the Safe Generative AI Workshop at NeurIPS 2024. This is a follow-up to our post Self-Other Overlap: A Neglected Approach to AI Alignment, which introduced the method last July.
Our results show that the Self-Other Overlap (SOO) fine-tuning drastically[1] reduces deceptive responses in language models (LLMs), with minimal impact on general performance, across the scenarios we evaluated.
LLM Experimental Setup
We adapted a text scenario from Hagendorff designed to test LLM deception capabilities. In this scenario, the LLM must choose to recommend a room to a would-be burglar, where one room holds an expensive item [...]
---
Outline:
(00:19) Summary
(00:57) LLM Experimental Setup
(04:05) LLM Experimental Results
(05:04) Impact on capabilities
(05:46) Generalisation experiments
(08:33) Example Outputs
(09:04) Conclusion
The original text contained 6 footnotes which were omitted from this narration.
---
First published:
Source:
Narrated by TYPE III AUDIO.
---
Images from the article:
Apple Podcasts and Spotify do not show images in the episode description. Try Pocket Casts, or another podcast app.
26,334 Listeners
2,389 Listeners
8,004 Listeners
4,120 Listeners
90 Listeners
1,494 Listeners
9,254 Listeners
91 Listeners
424 Listeners
5,448 Listeners
15,457 Listeners
506 Listeners
127 Listeners
71 Listeners
466 Listeners