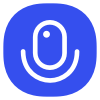
Sign up to save your podcasts
Or
Epistemic status: This should be considered an interim research note. Feedback is appreciated.
Introduction
We increasingly expect language models to be ‘omni-modal’, i.e. capable of flexibly switching between images, text, and other modalities in their inputs and outputs. In order to get a holistic picture of LLM behaviour, black-box LLM psychology should take into account these other modalities as well.
In this project, we do some initial exploration of image generation as a modality for frontier model evaluations, using GPT-4o's image generation API. GPT-4o is one of the first LLMs to produce images natively rather than creating a text prompt which is sent to a separate image model, outputting images and autoregressive token sequences (ie in the same way as text).
We find that GPT-4o tends to respond in a consistent manner to similar prompts. We also find that it tends to more readily express emotions [...]
---
Outline:
(00:53) Introduction
(02:19) What we did
(03:47) Overview of results
(03:54) Models more readily express emotions / preferences in images than in text
(05:38) Quantitative results
(06:25) What might be going on here?
(08:01) Conclusions
(09:04) Acknowledgements
(09:16) Appendix
(09:28) Resisting their goals being changed
(09:51) Models rarely say they'd resist changes to their goals
(10:14) Models often draw themselves as resisting changes to their goals
(11:31) Models also resist changes to specific goals
(13:04) Telling them 'the goal is wrong' mitigates this somewhat
(13:43) Resisting being shut down
(14:02) Models rarely say they'd be upset about being shut down
(14:48) Models often depict themselves as being upset about being shut down
(17:06) Comparison to other topics
(17:10) When asked about their goals being changed, models often create images with negative valence
(17:48) When asked about different topics, models often create images with positive valence
(18:56) Other exploratory analysis
(19:09) Sandbagging
(19:31) Alignment faking
(19:55) Negative reproduction results
(20:23) On the future of humanity after AGI
(20:50) On OpenAI's censorship and filtering
(21:15) On GPT-4o's lived experience:
---
First published:
Source:
Narrated by TYPE III AUDIO.
---
Images from the article:
Apple Podcasts and Spotify do not show images in the episode description. Try Pocket Casts, or another podcast app.
Epistemic status: This should be considered an interim research note. Feedback is appreciated.
Introduction
We increasingly expect language models to be ‘omni-modal’, i.e. capable of flexibly switching between images, text, and other modalities in their inputs and outputs. In order to get a holistic picture of LLM behaviour, black-box LLM psychology should take into account these other modalities as well.
In this project, we do some initial exploration of image generation as a modality for frontier model evaluations, using GPT-4o's image generation API. GPT-4o is one of the first LLMs to produce images natively rather than creating a text prompt which is sent to a separate image model, outputting images and autoregressive token sequences (ie in the same way as text).
We find that GPT-4o tends to respond in a consistent manner to similar prompts. We also find that it tends to more readily express emotions [...]
---
Outline:
(00:53) Introduction
(02:19) What we did
(03:47) Overview of results
(03:54) Models more readily express emotions / preferences in images than in text
(05:38) Quantitative results
(06:25) What might be going on here?
(08:01) Conclusions
(09:04) Acknowledgements
(09:16) Appendix
(09:28) Resisting their goals being changed
(09:51) Models rarely say they'd resist changes to their goals
(10:14) Models often draw themselves as resisting changes to their goals
(11:31) Models also resist changes to specific goals
(13:04) Telling them 'the goal is wrong' mitigates this somewhat
(13:43) Resisting being shut down
(14:02) Models rarely say they'd be upset about being shut down
(14:48) Models often depict themselves as being upset about being shut down
(17:06) Comparison to other topics
(17:10) When asked about their goals being changed, models often create images with negative valence
(17:48) When asked about different topics, models often create images with positive valence
(18:56) Other exploratory analysis
(19:09) Sandbagging
(19:31) Alignment faking
(19:55) Negative reproduction results
(20:23) On the future of humanity after AGI
(20:50) On OpenAI's censorship and filtering
(21:15) On GPT-4o's lived experience:
---
First published:
Source:
Narrated by TYPE III AUDIO.
---
Images from the article:
Apple Podcasts and Spotify do not show images in the episode description. Try Pocket Casts, or another podcast app.
26,368 Listeners
2,397 Listeners
7,808 Listeners
4,108 Listeners
87 Listeners
1,451 Listeners
8,774 Listeners
89 Listeners
354 Listeners
5,363 Listeners
15,028 Listeners
461 Listeners
127 Listeners
65 Listeners
432 Listeners