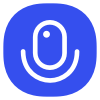
Sign up to save your podcasts
Or
When working through a problem, OpenAI's o1 model will write a chain-of-thought (CoT) in English. This CoT reasoning is human-interpretable by default, and I think that this is hugely valuable. Assuming we can ensure that these thoughts are faithful to the model's true reasoning, they could be very useful for scalable oversight and monitoring. I'm very excited about research to help guarantee chain-of-thought faithfulness.[1]
However, there's a impending paradigm for LLM reasoning that could make the whole problem of CoT faithfulness obsolete (and not in a good way). Here's the underlying idea, speaking from the perspective of a hypothetical capabilities researcher:
Surely human-interpretable text isn't the most efficient way to express thoughts. For every token that makes some progress towards the answer, you have to write a bunch of glue tokens like "the" and "is"—what a waste of time and compute! Many useful thoughts may even be inexpressible in [...]
---
Outline:
(03:50) Takeaways from the paper
(03:54) Training procedure
(04:54) Results
(06:39) Parallelized reasoning
(08:10) Latent reasoning can do things CoT cant
(10:18) COCONUT is not the literal worst for interpretability
(11:46) What can we do?
(11:56) Just... dont use continuous thoughts
(12:53) Government regulation
(13:43) Worst-case scenario: try to interpret the continuous thoughts
The original text contained 2 footnotes which were omitted from this narration.
---
First published:
Source:
Narrated by TYPE III AUDIO.
---
Images from the article:
Apple Podcasts and Spotify do not show images in the episode description. Try Pocket Casts, or another podcast app.
When working through a problem, OpenAI's o1 model will write a chain-of-thought (CoT) in English. This CoT reasoning is human-interpretable by default, and I think that this is hugely valuable. Assuming we can ensure that these thoughts are faithful to the model's true reasoning, they could be very useful for scalable oversight and monitoring. I'm very excited about research to help guarantee chain-of-thought faithfulness.[1]
However, there's a impending paradigm for LLM reasoning that could make the whole problem of CoT faithfulness obsolete (and not in a good way). Here's the underlying idea, speaking from the perspective of a hypothetical capabilities researcher:
Surely human-interpretable text isn't the most efficient way to express thoughts. For every token that makes some progress towards the answer, you have to write a bunch of glue tokens like "the" and "is"—what a waste of time and compute! Many useful thoughts may even be inexpressible in [...]
---
Outline:
(03:50) Takeaways from the paper
(03:54) Training procedure
(04:54) Results
(06:39) Parallelized reasoning
(08:10) Latent reasoning can do things CoT cant
(10:18) COCONUT is not the literal worst for interpretability
(11:46) What can we do?
(11:56) Just... dont use continuous thoughts
(12:53) Government regulation
(13:43) Worst-case scenario: try to interpret the continuous thoughts
The original text contained 2 footnotes which were omitted from this narration.
---
First published:
Source:
Narrated by TYPE III AUDIO.
---
Images from the article:
Apple Podcasts and Spotify do not show images in the episode description. Try Pocket Casts, or another podcast app.
26,331 Listeners
2,403 Listeners
7,873 Listeners
4,108 Listeners
87 Listeners
1,449 Listeners
8,765 Listeners
90 Listeners
354 Listeners
5,370 Listeners
15,300 Listeners
468 Listeners
128 Listeners
72 Listeners
438 Listeners