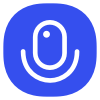
Sign up to save your podcasts
Or
4.9
6464 ratings
1,830 Listeners
1,459 Listeners
1,032 Listeners
519 Listeners
30,219 Listeners
194 Listeners
3,995 Listeners
1,757 Listeners
9,188 Listeners
9,095 Listeners
2,183 Listeners
28,304 Listeners
144 Listeners
1,341 Listeners
84 Listeners